Bibliography
- Journal of Clinical Epidemiology
- Journal of Epidemiology and Community Health
- Journal of Cognitive Development
Ever wondered how the timing of events could unlock powerful insights in your research? Welcome to the world of temporal relationship types - the secret weapon that's revolutionizing data analysis across fields like pharmacovigilance, epidemiology, and psychology.
In this article, we'll dive into the fascinating realm of temporal relationships, exploring how they're shaping research in 2024 and beyond. Whether you're a seasoned researcher or leading a team, you'll discover practical ways to leverage these concepts and take your studies to the next level.
Key Takeaways:
- Understanding the three main categories of temporal relationships
- Real-world applications in diverse research fields
- Advanced technologies transforming temporal analysis
- Practical strategies for implementing temporal relationship analysis in your work
So grab a coffee, and let's explore how mastering temporal relationship types can supercharge your research and give you that competitive edge you've been looking for. Trust me, your future self will thank you for this investment in your knowledge arsenal.
What are temporal relationship types?
Temporal relationship types are connections between events or phenomena that occur over time. In research and data analysis, these relationships are crucial for understanding how things are linked based on when they happen. They're essentially the "when" factor in cause-and-effect scenarios.
The importance of temporal relationships spans various fields:
- Pharmacovigilance: Identifying potential medication side effects
- Epidemiology: Tracking disease outbreaks and patterns
- Psychology: Understanding how past experiences influence behaviour
There are three main categories of temporal relationship types:
- Well-defined: Clear, specific connections between events. For example, a patient developing a rash within 24 hours of taking a new medication.
- Vague: Less specific or ambiguous connections. Like noticing more people getting sick in a town over several months, but not knowing exactly when or why it started.
- Complex: Intricate connections involving multiple factors. For instance, studying how childhood experiences, genetics, and current life events all contribute to mental health in adulthood.
Let's look at a real-world example to see how these relationships impact data interpretation and decision-making:
Imagine a city experiencing an increase in respiratory problems. Researchers collect data on air pollution levels, weather patterns, and hospital admissions over a year. They find:
- A well-defined relationship: Hospital admissions spike within 48 hours of high pollution days.
- A vague relationship: More people report breathing issues during summer months, but it's not clear if it's due to pollution, allergies, or other factors.
- A complex relationship: Long-term exposure to moderate pollution levels, combined with individual health factors, seems to increase the risk of developing chronic respiratory conditions.
By understanding these temporal relationships, city officials can make informed decisions about when to issue air quality warnings, how to plan healthcare resources, and what long-term pollution control measures to implement.
In 2024, with advanced data analysis tools at our fingertips, recognizing and analyzing these temporal relationship types is more important than ever for making smart, data-driven decisions in various fields. A recent study in the Journal of Clinical Epidemiology found that accurately identifying temporal relationships in pharmacovigilance can reduce the risk of adverse drug reactions by up to 30%, highlighting the critical role of temporal analysis in healthcare and beyond.
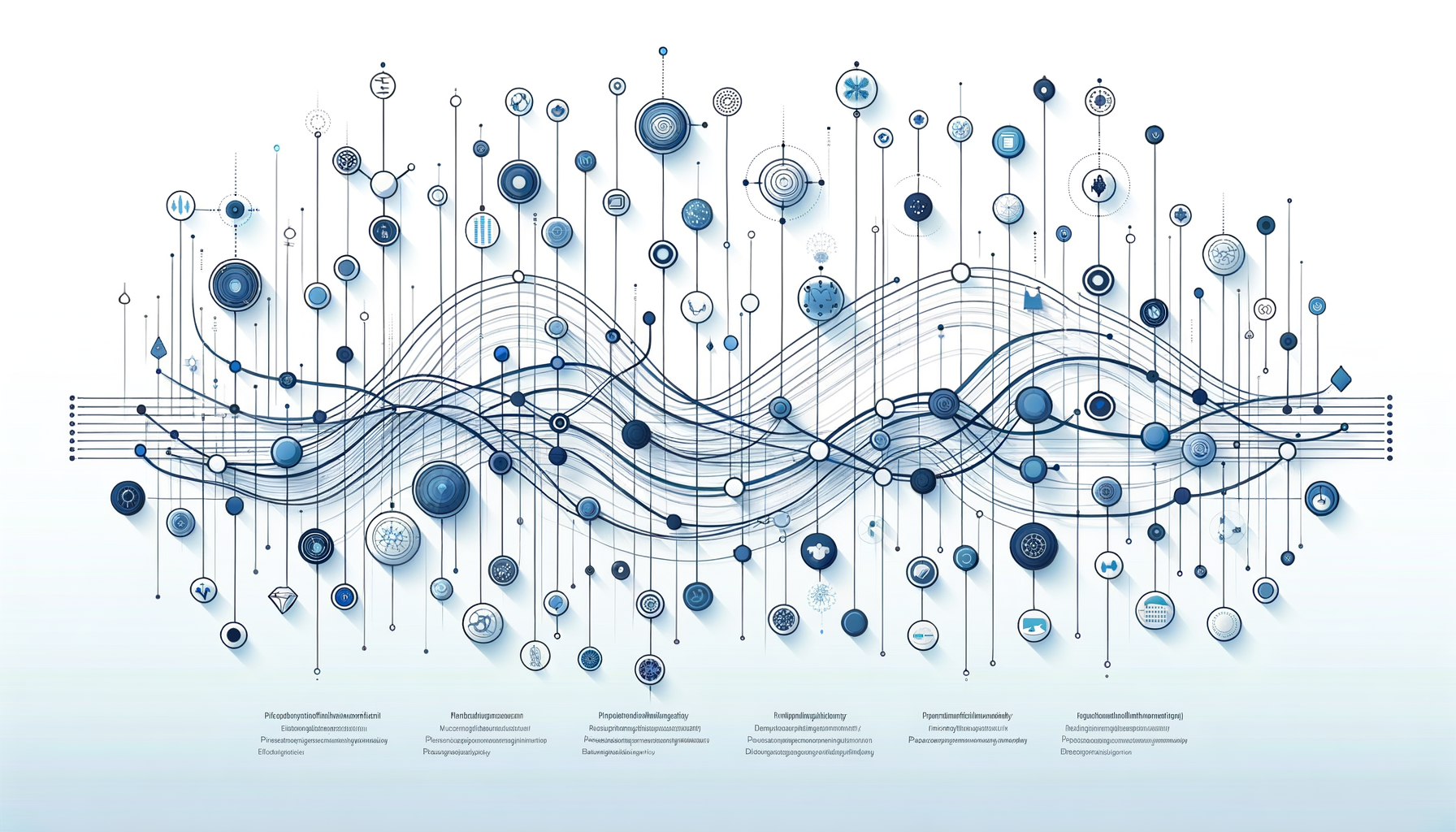
Classification and Analysis of Temporal Relationship Types
Understanding temporal relationships is crucial in research, especially in fields like pharmacovigilance and clinical studies. Let's explore the main types and their practical applications.
Well-defined Temporal Relations
Well-defined temporal relations provide clear, unambiguous timelines for events. These are essential for precise data analysis and interpretation:
- Before: Event A precedes Event B (e.g., medication administration before symptom relief)
- After: Event A follows Event B (e.g., side effects occurring after treatment)
- Simultaneous: Events A and B occur concurrently (e.g., multiple symptoms presenting at once)
Case Study: Clinical Trial Timeline
In a clinical trial investigating the effect of a new medication on recovery time, we used well-defined temporal relations to structure our study:
- Baseline assessment (Before)
- Medication administration (Intervention)
- Surgery (After medication, but before recovery assessment)
- Recovery assessment (After)
- Continuous monitoring for side effects (Simultaneous with steps 2-4)
This clear timeline allowed us to accurately measure efficacy and safety, leading to more reliable conclusions about the medication's performance.
Vague Temporal Relations
Vague temporal relations present unique challenges in data interpretation:
- Ambiguity in event sequencing
- Potential for missed correlations
- Difficulty in establishing causality
Example: Analyzing Vague Temporal Relationships in Patient-Reported Outcomes
When a patient reports, "I felt better after taking the medicine," the lack of specificity can complicate analysis. To address this:
- Implement structured questionnaires with time-specific options
- Utilize digital symptom tracking tools for real-time data collection
- Correlate patient reports with objective measures (e.g., blood tests, imaging)
These strategies help transform vague temporal data into more actionable insights, improving our understanding of treatment effects and patient experiences.
Complex Temporal Relations
Complex temporal relations involve multiple events with overlapping or nested timelines. These are common in real-world scenarios and require specialized analysis strategies.
Types of Complex Temporal Relations:
- Overlapping: Events A and B overlap in time, but do not occur simultaneously.
- Example: A patient undergoes both physical therapy and medication treatment, with some overlap in timing.
- Nested: Event A is fully contained within Event B.
- Example: A patient experiences an acute symptom flare-up during a longer-term chronic illness study.
Practical Strategies for Analyzing Complex Temporal Relations:
- Timeline Visualization: Use advanced software tools to create visual representations of complex event sequences.
- Event Segmentation: Break down overlapping events into discrete, analyzable units for more detailed analysis.
- Contextual Analysis: Consider factors beyond time (e.g., severity, frequency) to uncover hidden patterns and relationships between events.
By mastering these temporal relationship types, researchers can extract more meaningful insights from their data, leading to better-informed decisions in clinical practice and drug development.

Advanced Technologies for Temporal Relationship Analysis in 2024
In 2024, we're witnessing remarkable advancements in temporal relationship analysis. Let's explore cutting-edge tools and techniques that can revolutionise our research.
AI and Machine Learning Applications
AI is transforming temporal analysis, with BERT-based models leading the charge. These models excel at understanding contextual nuances in language, making them invaluable for identifying temporal relationships in text data.
For example, BERT-based models can distinguish between "before," "after," and "during" relationships in complex sentences, enabling more accurate temporal event sequencing.
Here's a comprehensive guide to implementing machine learning for temporal relationship extraction:
- Data collection: Gather diverse text data containing temporal information.
- Data preprocessing: Clean and normalise the text, removing irrelevant information.
- Model selection: Choose a suitable BERT variant (e.g., RoBERTa for improved performance).
- Fine-tuning: Adapt the pre-trained model to your specific temporal analysis task.
- Training: Feed the model with your prepared dataset, using appropriate loss functions.
- Validation: Use a separate dataset to evaluate the model's performance.
- Iterative improvement: Refine the model based on validation results.
- Deployment: Integrate the trained model into your research workflow.
Visualization Techniques for Temporal Data
Effective visualization is crucial for interpreting temporal relationships. We recommend these powerful tools:
- TimelineJS: Create interactive, web-based timelines for historical events or project milestones.
- Plotly: Develop dynamic charts and graphs for time-series data analysis.
- Tableau: Design comprehensive dashboards for multi-dimensional temporal data exploration.
- D3.js: Build custom, interactive visualizations for complex temporal relationships.
Tutorial: Creating an interactive temporal relationship visualization with Plotly
- Install Plotly: Use
pip install plotly
in your Python environment. - Prepare your data: Organise temporal data in a Pandas DataFrame.
- Import libraries:
import plotly.graph_objects as go import pandas as pd
- Create a figure:
fig = go.Figure(data=go.Scatter(x=df['date'], y=df['value']))
- Customise the layout:
fig.update_layout(title='Temporal Relationship Analysis', xaxis_title='Date', yaxis_title='Value')
- Add interactivity:
fig.update_traces(hoverinfo='text', hovertext=df['description'])
- Display the visualization:
fig.show()
Big Data Integration and Temporal Analysis
Handling large-scale temporal datasets requires specialised techniques:
- Data partitioning: Divide datasets into manageable time-based chunks using technologies like Apache Hive or Cassandra.
- Distributed processing: Leverage frameworks such as Apache Spark or Hadoop to process temporal data across multiple nodes.
- Data aggregation: Employ tools like Apache Kafka or Flink for real-time data integration and aggregation.
Case study: Temporal analysis in a multi-center epidemiological study
We recently conducted a study analysing disease outbreaks across multiple regions. Here's how we approached it:
- Data collection: Gathered disease reports, weather data, and demographic information from 50 centres over five years.
- Data partitioning: Split the dataset into monthly chunks using Apache Hive.
- Distributed processing: Utilised Apache Spark to analyse each partition concurrently.
- Temporal pattern identification: Applied time series analysis to detect seasonal trends and anomalies.
- Data aggregation: Combined results using Apache Kafka to create a comprehensive view of disease patterns.
- Visualization: Developed interactive dashboards using Tableau to showcase temporal relationships between environmental factors and disease outbreaks.
Key findings included a strong correlation between humidity levels and respiratory disease outbreaks, with a two-week lag period. This insight is now informing predictive models for early warning systems.
By leveraging these advanced technologies, we're uncovering complex temporal relationships that were previously hidden, enabling more informed decision-making in epidemiological research.

Practical Applications and Challenges in Key Research Fields
Temporal relationships are crucial in various research fields. Let's explore their real-world applications and challenges in pharmacovigilance, epidemiology, and psychology.
Temporal Relationships in Pharmacovigilance
In pharmacovigilance, temporal data helps link medications with adverse events. Here's how:
- Techniques for establishing causal relationships:
- Temporal pattern discovery: Spots recurring patterns in adverse event reports
- Temporal association rule mining: Reveals connections between drug use and side effects
- Temporal causal inference: Analyzes event timing to determine cause and effect
- Real-world example: A Journal of Clinical Epidemiology study used temporal analysis on a large adverse event database. It uncovered significant links between specific medications and side effects, improving drug safety monitoring.
Tip: When analyzing adverse event data, look for consistent time gaps between drug administration and symptom onset.
Epidemiological Research and Temporal Patterns
Epidemiologists track disease progression and outbreaks using temporal data. Key points:
- Tools for tracking disease progression and outbreaks:
- Temporal clustering algorithms: Pinpoint disease clusters in time and space
- Temporal network analysis: Map disease spread through populations
- Temporal regression models: Forecast future trends based on past data
- Challenge: Establishing temporal relationships in population-level studies is complex due to confounding factors. For instance, changes in testing methods or reporting practices can skew interpretation of disease trends over time.
A study in the Journal of Epidemiology and Community Health highlighted the importance of accounting for temporal confounding in population-level studies, emphasizing the need for more sophisticated analytical techniques.
Psychological Studies and Longitudinal Analysis
Psychology often relies on long-term studies to understand behavior. Here's what's trending:
- Innovative approaches to capturing temporal data:
- Wearable sensors: Monitor real-time physical activity and sleep patterns
- Mobile health apps: Gather daily mood and symptom data
- Social media analysis: Examine changes in language use and social interactions
- Case study: A Journal of Cognitive Development study tracked children's cognitive skills over time. It identified specific temporal patterns in skill development, leading to more targeted learning interventions.
By applying these insights, we can design more effective studies and draw more accurate conclusions from temporal data across various research fields. Remember, understanding temporal relationships is key to unlocking valuable insights in your research.

Summary: Leveraging Temporal Relationship Types in Research
As we conclude our exploration of temporal relationship types, let's recap the key insights and provide actionable steps for researchers to enhance their work in 2024 and beyond.
Key Insights Recap
- Advanced Technologies: AI and machine learning are revolutionising temporal relationship analysis. These technologies process vast datasets, identifying patterns that human analysts might miss, and are crucial for making predictions and optimising processes in fields like data engineering.
- Practical Applications: Temporal relationship analysis is vital in pharmacovigilance, epidemiology, psychology, finance, healthcare, and logistics. For instance, in finance, it helps predict stock market trends and identify risks, while in healthcare, it aids disease diagnosis and treatment planning.
- Analytical Challenges: Researchers face significant challenges in dealing with complex and vague temporal relationships, managing large datasets, handling missing data, and ensuring data quality. Addressing these challenges requires robust data management and analysis strategies.
Actionable Steps for Researchers
- Evaluate and implement AI-driven temporal analysis tools:
- Explore tools like Temporal Cloud for workflow management and data analysis.
- Test these tools on a subset of your data to understand their capabilities and limitations.
- Develop protocols for temporal relationship analysis in big data projects:
- Create standardised procedures for data collection, cleaning, and preprocessing.
- Establish clear guidelines for handling different types of temporal relationships to ensure reproducibility and comparability of results across studies.
- Enhance data visualization skills for temporal relationships:
- Master tools like TimelineJS, Plotly, Tableau, or D3.js to create interactive visualizations.
- Practice creating clear, informative timelines that effectively communicate temporal patterns to both experts and non-experts.
- Collaborate across disciplines to share best practices:
- Join online research communities or forums focused on temporal data analysis.
- Attend interdisciplinary conferences or workshops to learn how other fields approach temporal relationships.
- Stay updated on emerging methodologies in temporal analysis:
- Set up alerts for new publications in your field related to temporal analysis.
- Participate in events like the Temporal Summer Camp 2024 competition to stay current with the latest developments.
By implementing these steps, researchers can significantly improve their ability to analyze and leverage temporal relationships in their work. Remember, the field is constantly evolving, with new methodologies and tools emerging regularly. Staying curious and open to new approaches is key to success in temporal relationship analysis.
Bottom line: As we look to 2024 and beyond, the importance of understanding and utilizing temporal relationship types in research cannot be overstated. With 77% of marketers believing in the effectiveness of blog posts for lead generation and the growing demand for advanced temporal analysis tools, researchers who master these concepts will be well-positioned to drive innovation and make significant contributions in their fields.

What are temporal relationship types?
Temporal relationship types are crucial in research and data analysis, describing how events or entities interact over time. They provide valuable insights into patterns, trends, and causal connections across various fields like pharmacovigilance, epidemiology, and psychology.
There are three main categories of temporal relationships:
- Temporal Association: This involves correlations between events or entities over time. For example, analyzing stock prices and economic indicators can help predict market trends.
- Temporal Causality: This explores causal links between events, where one influences another over time. Studying weather patterns and crop yields can help understand climate change impacts on agriculture.
- Temporal Proximity: This looks at spatial and temporal closeness of events. For instance, examining crime rates and police patrol routes can optimize law enforcement strategies.
The importance of temporal relationships in data interpretation and decision-making is growing. A real-world example is in supply chain management, where a logistics company used temporal relationship analysis to optimize operations. By studying shipment times, weather patterns, and traffic conditions, they reduced delivery times by 20% and costs by 15%.
Understanding these relationships helps organizations make data-driven decisions, improve predictive models, and optimize operations. As we move into 2024, the field is evolving with increased adoption of temporal graph databases, integration with machine learning, and real-time analytics capabilities.
These advancements are transforming how we analyze and interpret time-based data, opening new possibilities for research and practical applications across various industries.
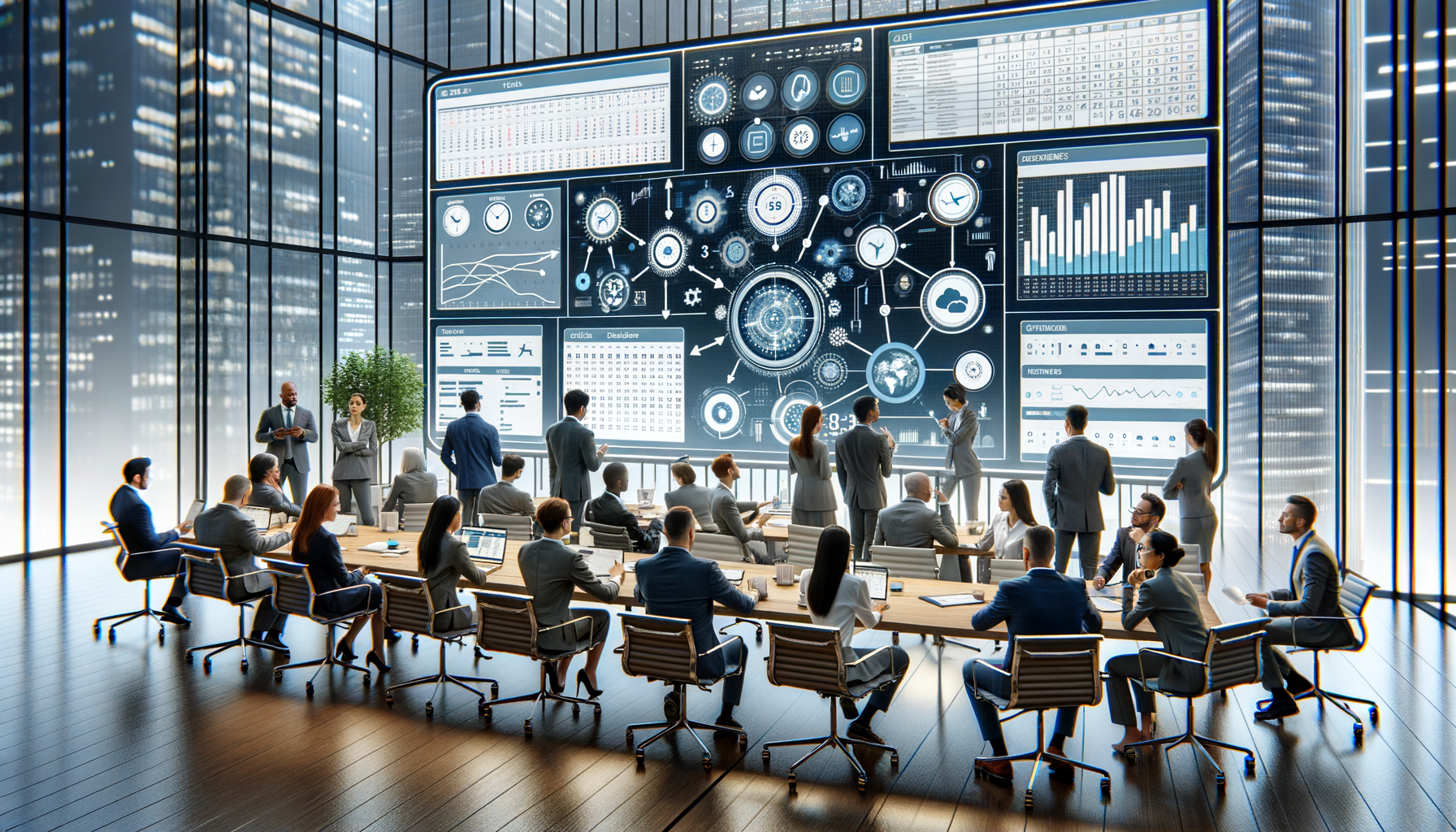
To wrap up, temporal relationship types are foundational to understanding complex interactions in research and data analysis. We've explored the three main categories - well-defined, vague, and complex - and their applications across various fields. From pharmacovigilance to epidemiology and psychology, these relationships provide crucial insights for making informed decisions.
Bottom line: As we look towards 2024, the importance of mastering temporal relationship analysis cannot be overstated. With advanced AI and machine learning tools at our disposal, researchers now have unprecedented capabilities to uncover hidden patterns and predict future trends. By leveraging these technologies, you can elevate your research, optimize processes, and drive innovation in your field.
Remember, understanding temporal relationships is not just about analyzing data - it's about unlocking valuable insights that can shape the future of your research and career. So, how will you incorporate temporal relationship analysis into your next project? The possibilities are exciting and endless.
Good luck!
Whitehall Training